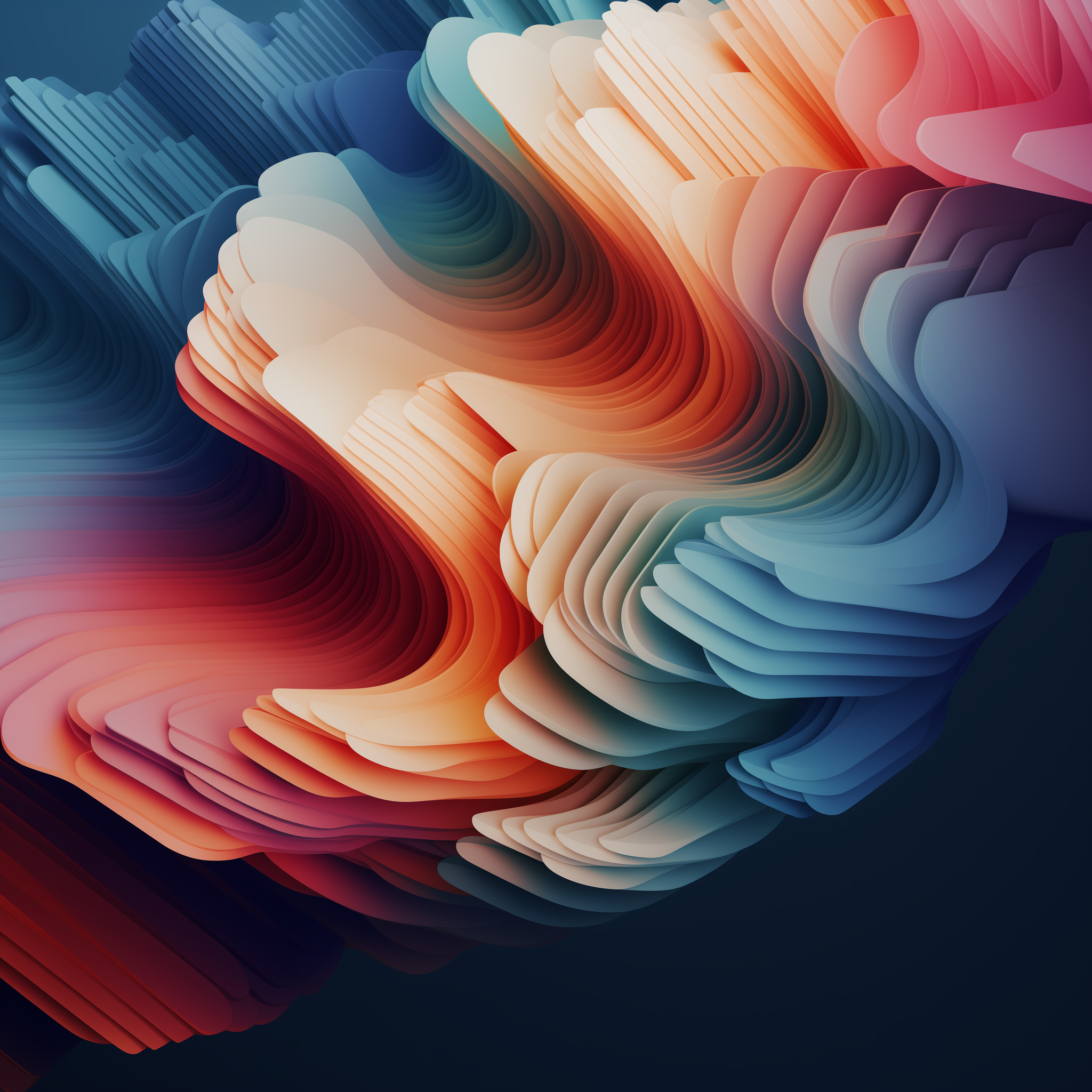
Collaboration at Scale: Unlocking Opportunity Through AI-Enabled Human Connection
I. Introduction: Why This Intelligence
In an era defined by global connectivity, access to opportunity remains strikingly unequal.
Traditional networking platforms optimize for attention rather than access. Despite living in a more digitally connected world than ever before, the ability to move upward economically still depends largely on who you know and whether you can reach them.
Nearly 5 billion people are connected online, yet upward mobility is declining. In the United States, a child born in the 1940s had a 90% chance of out-earning their parents; today, that number has fallen to just 50% (Chetty et al., 2016). Over 77% of jobs are filled through personal connections (LinkedIn, 2020), yet access to those networks is not evenly distributed—people from underrepresented backgrounds are three times more likely to report lacking the connections needed to achieve their goals (LinkedIn, 2020). This means that relationships—not just qualifications—are becoming the key to opportunity, making access to the right connections more critical than ever.
That’s why we built intelligence.com®: an AI model designed to enable users to share knowledge through trusted relationships. These connections—what sociologists call "weak ties"—are among the most powerful drivers of economic mobility. Yet most people don’t have the tools or transparency needed to unlock them.
This white paper outlines the case for a new type of intelligence—one that uses AI not to automate jobs or optimize ads, but to create economic value by sharing their networks. We call this Relationship Intelligence, and it’s built to give every person, regardless of background, a fairer shot at opportunity.
II. The Problem: Opaque Networks Limit Mobility
Access to opportunity is not just about talent or ambition—it’s about proximity to the right people at the right time. As Malcolm Gladwell pointed out in The Tipping Point: How Little Things Can Make a Big Difference, Connectors, people who excel at bringing people together and facilitating meaningful relationships, have massive social impact. intelligence.com enables every user to be a Connector by sharing who they know, with AI helping them find connections when needed.
Traditional networking tools favor the already connected. Those who lack the existing connections or the appearance of having them have less access to career mobility, funding, and knowledge. The algorithms driving recommendations are focused on volume of connections and reward users with large followings.
As a result, many people remain disconnected from life-changing introductions.
III. Why Relationships Matter: The Research Foundation
Not all connections are created equal. In 1973, sociologist Mark Granovetter published The Strength of Weak Ties, a now-seminal study that revealed a counterintuitive truth: when it comes to finding jobs and accessing opportunity, acquaintances—not close friends—are often more valuable (Granovetter, 1973).
Weak ties connect us to different networks. They offer access to novel information, referrals we wouldn’t otherwise get, and perspectives beyond our immediate circle. These second-degree connections—friends of friends—have been consistently shown to play an outsized role in economic mobility, innovation, and inclusion.
Granovetter’s insight laid the foundation for decades of research across multiple fields:
Economic Opportunity & Labor Markets
More than half of job seekers find work through weak social ties (Granovetter, 1995).
Those who span disconnected networks gain early access to new information and job opportunities (Burt, 1992).
Employee referrals from acquaintances improve hiring outcomes and speed (Fernandez & Weinberg, 1997).
Weak ties help people cross class and geographic boundaries to reach higher-opportunity roles (Lin et al., 1981).
Innovation, Knowledge Sharing & Entrepreneurship
Weak ties are ideal for transferring simple knowledge, while strong ties help convey complex insights (Hansen, 1999).
Innovation flourishes in networks with both embedded trust and external bridges (Uzzi, 1996; Obstfeld, 2005).
Entrepreneurs leverage diverse, loose connections to secure capital, legitimacy, and early traction (Stuart & Sorenson, 2007; Aldrich & Zimmer, 1986).
Digital Networks & Upward Mobility
In a groundbreaking study of Facebook’s network graph, researchers found that exposure to higher-income peers significantly increased upward mobility—especially for children in low-income communities (Chetty et al., 2022).
Weak ties on digital platforms are more likely to expose users to new job opportunities and diverse information (Bakshy et al., 2012; Ellison et al., 2007).
Bridging social capital—often created through weak ties—plays a critical role in increasing inclusion, especially in digitally-mediated networks (Putnam, 2000).
These findings converge on a single idea: opportunity is social—but the social graph is not equally accessible.
IV. A New Architecture for Opportunity
What if opportunity wasn’t locked behind closed networks? What if access could be revealed—ethically, transparently, and with permission?
The core premise behind intelligence.com is to build an AI-powered system to democratize access to high-value second-degree relationships.
At the heart of this model is a simple but powerful principle: people would be willing to share who they know in exchange for shared value.
Our Architecture is Rooted in Three Core Principles:
1. Permissioned Transparency
Users give permission to share information about their real-world relationships to unlock value—transparently and with purpose. The AI helps infer possible second-degree ties and then confirms accuracy with human input.
2. AI that Reveals Likely Value
Our model learns from signal-rich data: shared professional context, historical introductions, mutual connections who’ve helped others succeed. This prioritization of value over volume makes the system radically more useful than traditional social networks.
3. Equity Through Shared Access
Participants don’t need massive followings or elite networks to benefit. Instead, they contribute who they truly know—and in return, gain visibility into others’ relationships. This creates a level playing field: a trust-based exchange of social capital that can unlock introductions, advice, funding, jobs, and more.
This is a different kind of network: an infrastructure of mutual access where AI helps users find and fuel each other’s success.
V. Engineering the System for Trust
How It Works:
When users join intelligence.com, they opt into a cooperative relationship graph—signaling their willingness to build trusted connections and help others. The system uses this permission to surface likely shared relationships, always with transparency and purpose. The system learns from historical data, interaction patterns, and mutual relationships to suggest potential helpful ties.
Some examples of where having access is highly valuable include:
Career transitions: Discovering introductions that would otherwise be invisible.
Resource-sharing: Finding people who can offer advice, insight, or support during critical moments.
Access to capital: Identifying shared relationships who can unlock funding, customers, or early believers.
Social capital becomes a renewable resource—one that can be distributed more equitably.
VI. Why This Capability, Why Now
Relationship Intelligence is the foundation of opportunity. In today’s world, talent is abundant—but access is not. People still lack visibility into the networks that could unlock their potential.
We’re training AI to surface timely, trusted relationships that open doors to opportunity. These connections are relevant, permissioned, and backed by a growing body of research showing that economic mobility depends not just on what you know, but who you can reach.
This capability is essential infrastructure for equitable growth.
Why Now?
We have reached a breaking point:
Mobility is stalling. Only 50% of people today are expected to earn more than their parents, compared to 90% in the 1940s (Chetty et al., 2016). The promise of upward mobility is no longer guaranteed—it must be rebuilt.
Networks shape outcomes. Research consistently shows that weak ties—connections between people who don’t know each other directly—are the most effective paths to jobs, funding, and economic advancement (Granovetter, 1973; Rajkumar et al., 2022).
Relationships across class lines change lives. Economic connectedness—the degree to which people have relationships that span income levels—is the single strongest predictor of upward mobility in the United States (Chetty et al., 2022). These are the kinds of ties most people don’t realize they already have—or could access.
Technology can now make the invisible visible. Advances in graph neural networks, reinforcement learning, and behavioral modeling make it possible to surface high-value connections that would otherwise remain hidden—ethically, intelligently, and at scale.
Relationship Intelligence offers a path forward: a way to design systems around connection, trust, and access—not just speed or scale.
Why This Model?
Because it reflects the science, supports the user, and meets the moment.
1. It’s rooted in decades of proven research.
Weak ties create more economic mobility than close personal networks (Granovetter, 1973).
Bridging social divides—economic connectedness—is the best predictor of upward mobility (Chetty et al., 2022).
People who span structural holes gain strategic advantage and unlock information flows (Burt, 1992).
This model activates those insights by making relationships actionable and discoverable—within a system people can trust.
2. It’s designed to scale trust.
The system learns from permissioned relationships, behavioral context, and shared goals—not from volume or visibility. Built using advanced graph-based intelligence, it’s designed to:
Identify the most relevant connections
Prioritize quality and mutual benefit
Surface trusted introductions when and where they’re needed most
Uphold transparency and user control at every step
This is a new kind of intelligence—one that measures what matters: shared trust, potential value, and timing.
3. It creates value at every level.
For individuals, the model helps unlock introductions, mentorship, capital, and opportunity.
For organizations and economies, it builds inclusive infrastructure for talent, innovation, and long-term growth.
This is more than a model. It’s a movement toward a more connected, more equitable future.
A future where every person—regardless of background—can access the relationships they need to thrive.
VII. Technical Appendix
This section provides a high-level overview of how intelligence.com maps relationships, powers recommendations, and ensures responsible AI design.
At the core of our relationship intelligence system lies a Graph Neural Network (GNN) architecture that enables dynamic, contextual, and interpretable modeling of interpersonal relationships. This system goes far beyond legacy models that treat connections as static and uniformly important. Instead, we model relationships as evolving networks of trust, communication, and strategic value, enabling precise predictions and tailored recommendations.
1. Graph Construction: Modeling the Real Relationship Landscape
We build a heterogeneous, attributed graph where each node represents an individual (e.g., buyer, seller, teammate), and each edge represents a meaningful interaction or inferred connection.
Node Attributes (Individuals):
We construct rich profiles of individuals using a blend of organizational context, behavioral signals, and communication patterns. Attributes may include role-related metadata, interaction tendencies, and inferred engagement profiles that help characterize influence, responsiveness, and relevance within a business context.
Edge Attributes (Connections):
Relationships between individuals are modeled based on multi-channel interaction patterns, including communication recency, consistency, and qualitative tone. Connections are further enriched with contextual indicators that reflect trust, collaboration potential, and business relevance—augmented by relevant deal or opportunity metadata when applicable.
This graph captures not just who knows whom, but how strong, trust-based, or transactional that relationship is—derived directly from real behavior and communication patterns.
2. GNN Architecture: GraphSAGE for Scalable, Inductive Learning
To model complex and dynamic graph structures at scale, we deploy GraphSAGE (Graph Sample and Aggregate), a state-of-the-art inductive GNN that generalizes to unseen nodes and graphs—perfect for evolving CRMs.
Key Components:
Aggregation Techniques:
Mean Aggregator to capture general trends
LSTM Aggregator for sequence-aware feature aggregation
Pooling Aggregator for higher-level summarization
GCN Aggregator to leverage neighborhood similarity
Fixed-Size Neighbor Sampling: Efficiently processes large, sparse graphs
Training Mode:
Unsupervised node embedding using contrastive learning
Negative sampling to distinguish meaningful relationships from noise
Optimization using triplet loss or sampled softmax
Evaluation Tools:
t-SNE for embedding visualization
Silhouette score for clustering quality
Graph metrics (e.g., degree centrality, betweenness, closeness) to identify key influencers and bridge nodes
3. Applications & Outputs: Turning Graph Intelligence into Action
The embeddings and insights generated by the GNN power a wide array of downstream applications, all tied to CRM value creation:
Connector Recommendations: Identify the best internal champions for a given buyer or seller based on relational proximity, trustworthiness, and communication quality.
Relationship Scoring: Compute fine-grained relationship scores that reflect strength, intent, trust, and influence, enabling smarter outreach and prioritization.
Similarity & Clustering: Group users into dynamic clusters based on behavioral and relational similarity, enabling:
Lookalike modeling for prospects
Automated team-matching
Strategic coverage analysis
Network Mapping & Influence Analytics: Visualize relationship networks and compute key graph-theoretic features to understand:
Influence pathways within and across organizations
Central nodes (connectors, brokers, gatekeepers)
Risk of communication silos or over-reliance on single individuals
Responsible AI: Controls and Safeguards
Every part of the system is built to preserve trust and enforce transparency:
Fairness and Bias Mitigation: Models are monitored and evaluated across demographic groups to ensure equitable representation and outcomes.
Built for Future Transparency: We’re designing the system so users will be able to see why a relationship was recommended and have full control to confirm, remove, or opt out.
Differential Privacy (Planned): To further strengthen data protections, privacy-preserving techniques are being explored to ensure training happens on aggregate patterns, not individual records.
System Roadmap
As the model evolves, the technical team will continue to refine:
Edge weighting models based on user goals and connection outcomes
Natural language understanding for relationship context inference (with opt-in permissions)
Open API integrations for secure, cross-platform relationship graph enrichment
This architecture reflects a deliberate choice: to build AI that respects, strengthens, and responsibly reveals human relationships.
Click here for works cited.